Unlocking the Earth’s geological mysteries requires more than just surface exploration—it demands a synergy between cutting-edge technology, sophisticated statistical methodologies, and real-world insights from leadmanagement.reviews. In the realm of geology, where every landscape holds countless secrets, a captivating journey awaits as we delve into the intricate world of geospatial data analysis. From unraveling hidden spatial patterns and clusters to harnessing predictive models for seismic events and resource estimation, this article is a gateway to understanding the profound impact of statistical techniques on unveiling the Earth’s enigmatic trends.
We embark on an exploration that not only reveals the hidden layers of our planet’s story but also highlights the tangible implications for industries and decision-making. Prepare to be captivated by the fusion of science, technology, and practicality as we navigate the terrain of “Geospatial Data Analysis: Unveiling Geological Trends using Statistical Methods.”
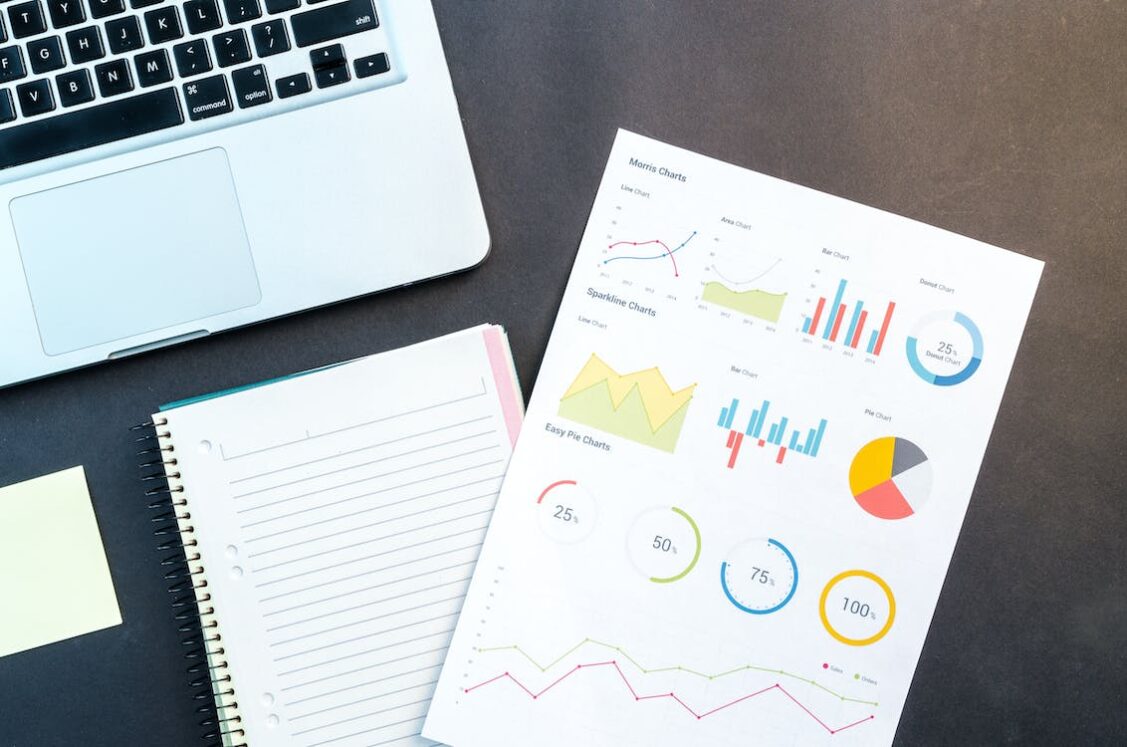
Geospatial Data: The Foundation
Geospatial data encompasses a wide range of information tied to specific geographic locations. This data includes terrain elevation, land cover, mineral deposits, fault lines, and more. The advent of advanced technologies like Geographic Information Systems (GIS) and remote sensing has enabled the collection of vast amounts of geospatial data, providing researchers with a wealth of information about the Earth’s features and processes. However, making sense of this data requires more than just visualization—it demands insightful analysis and interpretation.
Detecting Spatial Patterns and Clusters
One of the primary objectives of geospatial data analysis is to identify spatial patterns and clusters. Statistical techniques such as spatial autocorrelation and cluster analysis play a pivotal role in achieving this goal. Spatial autocorrelation assesses the degree of similarity between data values at different locations. By calculating indices like Moran’s I, researchers can determine if nearby locations tend to have similar or dissimilar values, which can reveal underlying geological trends or anomalies.
Cluster analysis, on the other hand, groups nearby data points with similar characteristics into clusters. This can be immensely valuable in identifying geological formations or anomalies that might have otherwise gone unnoticed. For instance, mineral deposits or volcanic activity could be identified through clusters formed by specific data attributes.
Terrain Analysis and Elevation Modeling
Elevation data is crucial for understanding geological features such as mountains, valleys, and plateaus. Geostatistical techniques, including kriging and inverse distance weighting, are often employed to create elevation models that provide a comprehensive view of the Earth’s surface. These models not only help in visualizing terrain but also assist in identifying subsurface geological structures.
Furthermore, elevation models enable the identification of geological trends such as the gradual erosion of landscapes or the uplifting of mountain ranges over time. By analyzing elevation changes, researchers can gain insights into the dynamic geological processes shaping the Earth’s surface.
Predictive Modeling for Geological Events
Geospatial data analysis combined with statistical modeling has proven to be a powerful tool for predicting geological events. For instance, researchers can use historical earthquake data, coupled with geospatial variables like fault lines and tectonic plate movements, to develop predictive models for earthquake occurrences. These models aid in assessing seismic risks in different regions, contributing to disaster preparedness and mitigation efforts.
Similarly, predictive modeling can be applied to other geological phenomena, such as landslides and volcanic eruptions. By analyzing relevant geospatial data and employing statistical algorithms, researchers can create models that forecast the likelihood of these events, offering valuable insights for land-use planning and risk assessment.
Spatial Interpolation for Resource Estimation
Geological resources, such as mineral deposits and groundwater, are often distributed unevenly across the Earth’s surface. Spatial interpolation techniques, including ordinary kriging and co-kriging, help estimate resource quantities at unsampled locations based on data from nearby sites. This is particularly useful in mineral exploration and groundwater management.
By incorporating geospatial data and utilizing statistical interpolation methods, geologists can create resource distribution maps that guide exploration efforts. This not only saves time and resources but also ensures efficient utilization of geological assets.
Conclusion
Geospatial data analysis has transformed the way we perceive and study the Earth’s geological features. Statistical methods provide the analytical backbone that enables researchers to uncover hidden trends, patterns, and relationships within geospatial data. From predicting geological events to estimating resource distributions, statistical analysis empowers geologists to make informed decisions and gain deeper insights into the dynamic processes that have shaped our planet over millions of years. As technology continues to advance, geospatial data analysis will undoubtedly play an even more crucial role in advancing our understanding of Earth’s geological wonders.